Archive for the ‘Stat’ Category.
Jul 25th, 2007| 02:28 am | Posted by hlee
Since I began to subscribe arxiv/astro-ph abstracts, from an astrostatistical point of view, one of the most frequent topics has been photometric redshifts. This photometric redshift has been a popular topic as the catalog of remote photometric object observation multiplies its volume and sky survey projects in multiple bands lead to virtual observatories (VO – will discuss in the later posting). Just searching by photometric redshifts in google scholar and arxiv.org provides more than 2000 articles since 2000.
Continue reading ‘Photometric Redshifts’ »
Tags:
cosmology,
distance estimation,
Lutz-Kelker bias,
machine learning,
Malmquist bias,
Photometric Redshift,
spectrum,
survey,
VO Category:
Algorithms,
arXiv,
Data Processing,
Galaxies,
Stat |
Comment
Jul 19th, 2007| 11:01 pm | Posted by aconnors
Ten years ago, Astrophysicist John Nousek had this answer to Hyunsook Lee’s question “What is so special about chi square in astronomy?”:
The astronomer must also confront the problem that results need to be published and defended. If a statistical technique has not been widely applied in astronomy before, then there are additional burdens of convincing the journal referees and the community at large that the statistical methods are valid.
Certain techniques which are widespread in astronomy and seem to be accepted without any special justification are: linear and non-linear regression (Chi-Square analysis in general), Kolmogorov-Smirnov tests, and bootstraps. It also appears that if you find it in Numerical Recipes (Press etal. 1992) that it will be more likely to be accepted without comment.
…Note an insidious effect of this bias, astronomers will often choose to utilize a widely accepted statistical tool, even into regimes where the tool is known to be invalid, just to avoid the problem of developping or researching appropriate tools.
From pg 205, in “Discussion by John Nousek” (of Edward J. Wegman et. al., “Statistical Software, Siftware, and Astronomy”), in Statistical Challenges in Modern Astronomy II”, editors G. Jogesh Babu and Eric D. Feigelson, 1997, Springer-verlag, New York.
Jul 16th, 2007| 03:31 pm | Posted by hlee
From arxiv/astro-ph:0707.2064v1
Star Formation via the Little Guy: A Bayesian Study of Ultracool Dwarf Imaging Surveys for Companions by P. R. Allen.
I rather skip all technical details on ultracool dwarfs and binary stars, reviews on star formation studies, like initial mass function (IMF), astronomical survey studies, which Allen gave a fair explanation in arxiv/astro-ph:0707.2064v1 but want to emphasize that based on simple Bayes’ rule and careful set-ups for likelihoods and priors according to data (ultracool dwarfs), quite informative conclusions were drawn:
Continue reading ‘[ArXiv] Bayesian Star Formation Study, July 13, 2007’ »
Tags:
Bayesian,
binary,
dwarfs,
IMF,
likelihood,
prior,
star formation,
survey,
upper limit Category:
arXiv,
Bayesian,
Objects |
1 Comment
Jul 16th, 2007| 01:30 pm | Posted by hlee
From arxiv/astro-ph:0707.1982v1,
Nflation: observable predictions from the random matrix mass spectrum by Kim and Liddle
To my knowledge, random matrix received statisticians’ interests fairly recently and SAMSI (Statistical and Applied Mathematical Sciences Institute) offered a semester long program on High Dimensional Inference and Random Matrices (tutorials and lecture notes can be found) during Fall 2006 . However, my knowledge is very limited to make a comment or critic on Kim and Liddle’s paper. Clearly, nonetheless, this paper is not about random matrix theory but about its straightforward application to the cosmological model viability.
Continue reading ‘[ArXiv] Random Matrix, July 13, 2007’ »
Jul 13th, 2007| 07:24 pm | Posted by hlee
From arxiv/astro-ph: 0707.1611 Probabilistic Cross-Identification of Astronomical Sources by Budavari and Szalay
As multi-wave length studies become more popular, various source matching methodologies have been discussed. One of such methods particularly focused on Bayesian idea was introduced by Budavari and Szalay with a demand for symmetric algorithms in a unified framework.
Continue reading ‘[ArXiv] Matching Sources, July 11, 2007’ »
Tags:
Bayes factor,
evidence,
Matching,
multi-wavelength,
Multiple Testing Category:
Algorithms,
arXiv,
Bayesian,
Data Processing,
Frequentist,
Objects,
Quotes,
Uncertainty |
1 Comment
Jul 12th, 2007| 03:37 pm | Posted by aconnors
This is from the very interesting Ingrid Daubechies interview by Dorian Devins,
www.nasonline.org/interviews_daubechies, National Academy of Sciences, U.S.A., 2004. It is from part 6, where Ingrid Daubechies speaks of her early mathematics paper on wavelets. She tries to put the impact into context:
I really explained in the paper where things came from. Because, well, the mathematicians wouldn’t have known. I mean, to them this would have been a question that really came out of nowhere. So, I had to explain it …
I was very happy with [the paper]; I had no inkling that it would take off like that… [Of course] the wavelets themselves are used. I mean, more than even that. I explained in the paper how I came to that. I explained both [a] mathematicians way of looking at it and then to some extent the applications way of looking at it. And I think engineers who read that had been emphasizing a lot the use of Fourier transforms. And I had been looking at the spatial domain. It generated a different way of considering this type of construction. I think, that was the major impact. Because then other constructions were made as well. But I looked at it differently. A change of paradigm. Well, paradigm, I never know what that means. A change of … a way of seeing it. A way of paying attention.
Jul 5th, 2007| 04:13 pm | Posted by aconnors
Jeff Scargle (in person [top] and in wavelet transform [bottom], left) weighs in on our continuing discussion on how well “automated fitting”/”Machine Learning” can really work (private communication, June 28, 2007):
It is clearly wrong to say that automated fitting of models to data is impossible. Such a view ignores progress made in the area of machine learning and data mining. Of course there can be problems, I believe mostly connected with two related issues:
* Models that are too fragile (that is, easily broken by unusual data)
* Unusual data (that is, data that lie in some sense outside the arena that one expects)
The antidotes are:
(1) careful study of model sensitivity
(2) if the context warrants, preprocessing to remove “bad” points
(3) lots and lots of trial and error experiments, with both data sets that are as realistic as possible and ones that have extremes (outliers, large errors, errors with unusual properties, etc.)
Trial … error … fix error … retry …
You can quote me on that.
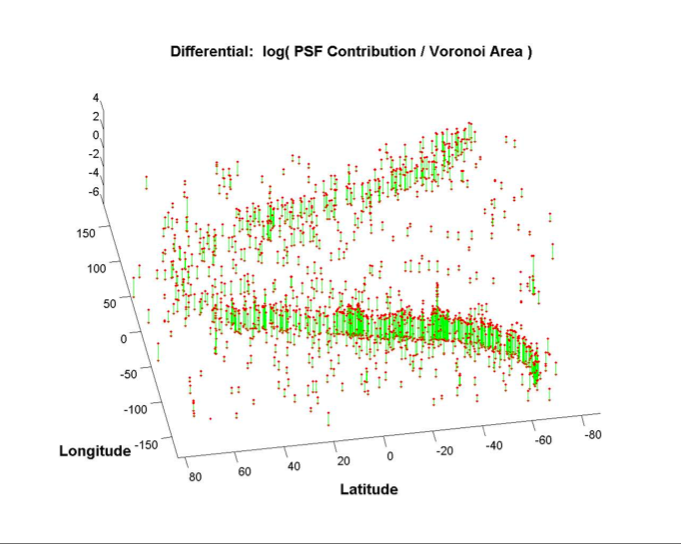
This ilustration is from Jeff Scargle’s First GLAST Symposium (June 2007) talk, pg 14, demonstrating the use of inverse area of Voroni tesselations, weighted by the PSF density, as an automated measure of the density of Poisson Gamma-Ray counts on the sky.
Category:
Algorithms,
Astro,
Data Processing,
gamma-ray,
High-Energy,
Imaging,
Methods,
Quotes,
Stat,
Timing,
X-ray |
1 Comment
Jul 2nd, 2007| 06:07 pm | Posted by hlee
From arXiv/astro-ph:0706.4484
Spectroscopic Surveys: Present by Yip. C. overviews recent spectroscopic sky surveys and spectral analysis techniques toward Virtual Observatories (VO). In addition that spectroscopic redshift measures increase like Moore’s law, the surveys tend to go deeper and aim completeness. Mainly elliptical galaxy formation has been studied due to more abundance compared to spirals and the galactic bimodality in color-color or color-magnitude diagrams is the result of the gas-rich mergers by blue mergers forming the red sequence. Principal component analysis has incorporated ratios of emission line-strengths for classifying Type-II AGN and star forming galaxies. Lyα identifies high z quasars and other spectral patterns over z reveal the history of the early universe and the characteristics of quasars. Also, the recent discovery of 10 satellites to the Milky Way is mentioned.
Continue reading ‘[ArXiv] Spectroscopic Survey, June 29, 2007’ »
Tags:
bimodality,
chi-square minimization,
Classification,
CMD,
Estimation,
machine learning,
massive data,
model based,
PCA,
spectral analysis,
spectroscopic,
survey,
VO Category:
arXiv,
Astro,
Bayesian,
Data Processing,
Fitting,
Frequentist,
Methods,
Spectral |
Comment
Jun 28th, 2007| 01:01 am | Posted by aconnors
I want to use this short quote by Andrew Gelman to highlight many interesting topics at the recent Third Workshop on Monte Carlo Methods. This is part of Andrew Gelman’s empahsis on the fundamental importance of thinking through priors. He argues that “non-informative” priors (explicit, as in Bayes, or implicit, as in some other methods) can in fact be highly constraining, and that weakly informative priors are more honest. At his talk on Monday, May 14, 2007 Andrew Gelman explained:
You want to supply enough structure to let the data speak,
but that’s a tricky thing.
Jun 27th, 2007| 02:23 pm | Posted by hlee
From arXiv:physics.data-an/0706.3622v1:
Comments on the unified approach to the construction of classical confidence intervals
This paper comments on classical confidence intervals and upper limits, as the so-called a flip-flopping problem, both of which are related asymptotically (when n is large enough) by the definition but cannot be converted from one to the another by preserving the same coverage due to the poisson nature of the data.
Continue reading ‘[ArXiv] Classical confidence intervals, June 25, 2007’ »
Jun 25th, 2007| 01:27 pm | Posted by hlee
One of the papers from arxiv/astro-ph discusses kernel regression and model selection to determine photometric redshifts astro-ph/0706.2704. This paper presents their studies on choosing bandwidth of kernels via 10 fold cross-validation, choosing appropriate models from various combination of input parameters through estimating root mean square error and AIC, and evaluating their kernel regression to other regression and classification methods with root mean square errors from literature survey. They made a conclusion of flexibility in kernel regression particularly for data at high z.
Continue reading ‘[ArXiv] Kernel Regression, June 20, 2007’ »
Tags:
AIC,
BIC,
Classification,
cross-validation,
kernel,
photometric redshifts,
regression,
SDSS Category:
arXiv,
Frequentist,
Galaxies,
Stat |
Comment
Jun 20th, 2007| 06:29 pm | Posted by aconnors
These quotes are in the opposite spirit of the last two Bayesian quotes.
They are from the excellent “R”-based , Tutorial on Non-Parametrics given by
Chad Schafer and Larry Wassserman at the 2006 SAMSI Special Semester on AstroStatistics (or here ).
Chad and Larry were explaining trees:
For more sophistcated tree-searches, you might try Robert Nowak [and his former student, Becca Willett --- especially her "software" pages]. There is even Bayesian CART — Classifcation And Regression Trees. These can take 8 or 9 hours to “do it right”, via MCMC. BUT [these results] tend to be very close to [less rigorous] methods that take only minutes.
Trees are used primarily by doctors, for patients: it is much easier to follow a tree than a kernel estimator, in person.
Trees are much more ad-hoc than other methods we talked about, BUT they are very user friendly, very flexible.
In machine learning, which is only statistics done by computer scientists, they love trees.
Jun 19th, 2007| 05:00 pm | Posted by hlee
From arxiv/astro-ph, arXiv:0706.2590v1 Extreme Value Theory and the Solar Cycle by Ramos, A. This paper might drag a large attention from CHASC members.
Continue reading ‘[ArXiv] Solar Cycle, June 18, 2007’ »
Jun 13th, 2007| 05:51 pm | Posted by aconnors
This is the second a series of quotes by
Xiao Li Meng , from an introduction to Markov Chain Monte Carlo (MCMC), given to a room full of astronomers, as part of the April 25, 2006 joint meeting of Harvard’s “Stat 310″ and the California-Harvard Astrostatistics Collaboration. This one has a long summary as the lead-in, but hang in there!
Summary first (from earlier in Xiao Li Meng’s presentation):
Let us tackle a harder problem, with the Metropolis Hastings Algorithm.
An example: a tougher distribution, not Normal in [at least one of the dimensions], and multi-modal… FIRST I propose a draw, from an approximate distribution. THEN I compare it to true distribution, using the ratio of proposal to target distribution. The next draw: tells whether to accept the new draw or stay with the old draw.
Our intuition:
1/ For original Metropolis algorithm, it looks “geometric” (In the example, we are sampling “x,z”; if the point falls under our xz curve, accept it.)
2/ The speed of algorithm depends on how close you are with the approximation. There is a trade-off with “stickiness”.
Practical questions:
How large should say, N be? This is NOT AN EASY PROBLEM! The KEY difficulty: multiple modes in unknown area. We want to know all (major) modes first, as well as estimates of the surrounding areas… [To handle this,] don’t run a single chain; run multiple chains.
Look at between-chain variance; and within-chain variance. BUT there is no “foolproof” here… The starting point should be as broad as possible. Go somewhere crazy. Then combine, either simply as these are independent; or [in a more complicated way as in Meng and Gellman].
And here’s the Actual Quote of the Week:
[Astrophysicist] Aneta Siemiginowska: How do you make these proposals?
[Statistician] Xiao Li Meng: Call a professional statistician like me.
But seriously – it can be hard. But really you don’t need something perfect. You just need something decent.
Jun 13th, 2007| 01:27 pm | Posted by hlee
A Link to Statistical Analysis for the Virtual Observatory is added. Its description with toy data is given at
http://www.astrostatistics.psu.edu/vostat/.
Nice feature of this website is that the interface allows you to perform various statistical analysis on a data set which is located either at your hard disk or at the Virtual Observatory.